AI Dragon Approach
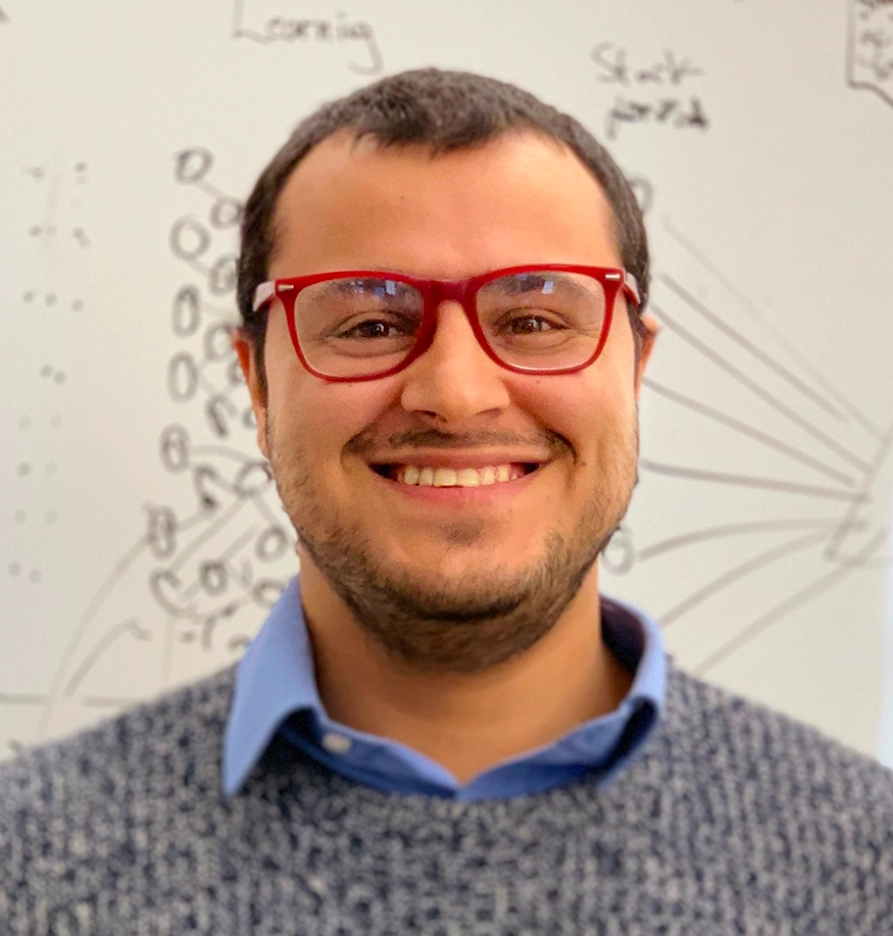
March 2025
Broad Approach
Unlike approaches reliant on specific hypotheses or individual data points, Portfolio Dragon employs a strategy of broad generalization. Our AI engine is designed not to be pre-programmed with specific market theories but rather to learn from the vast complexity of the financial markets themselves. This allows the engine to uncover previously unseen patterns and relationships, adapting to evolving market dynamics and generating highly robust and adaptable predictions.
It's crucial to understand that the AI signal generated by Portfolio Dragon's engine is carefully designed and optimized for seamless integration with our proprietary portfolio construction and risk engine. This integration is vital because it allows the signal to work in concert with other factors such as risk management constraints, investment objectives and portfolio rebalancing strategies. By embedding the AI signal within this broader framework, we ensure that investment decisions are not only informed by predicted performance but also by the overall portfolio's strategic goals and risk tolerance, generating optimal, well-diversified portfolios tailored to your specific needs. This holistic approach provides a considerable advantage over standalone signal-based strategies.
Four Key Steps
1. Data Ingestion
The engine's design incorporates daily data updates for 45,000+ assets and draws from diverse sources (macroeconomic, fundamental, market, commodity among others) to create a robust and comprehensive dataset. This approach is crucial because it ensures responsiveness to current market events and allows for capturing the complex interplay of factors influencing asset prices, leading to more accurate and timely forecasts than would be possible with less frequent updates or a narrower data scope. The engine is trained on 22+ billion financial data points.
2. Feature Engineering
The generation of 400+ features from the ingested data aims to capture subtle, predictive patterns. The use of auto-encoding enhances this process by denoising the data, reducing dimensionality, and identifying crucial predictive information in a compressed representation. This approach is not only effective in improving forecasting accuracy but is also crucial for efficiency in handling the enormous volume of data involved.
3. Ensemble Model Architecture
The engine utilizes an ensemble of four distinct machine learning model families to create a robust and adaptable forecasting system. This diverse approach mitigates the risk of overfitting and underperformance associated with relying on a single model type, by leveraging the strengths of each. Quarterly retraining ensures the model remains current, adapting to changing market conditions and reducing forecast degradation over time, while the ensembling of their results further enhances prediction accuracy and efficiency.
4. Signal Generation
The AI engine produces a daily probabilistic signal, forecasting the likelihood of price movements within the next month. This probabilistic approach allows for a more nuanced understanding of risk, providing investors with a measure of confidence in the forecast.