Crowding and Market Impact from AI Integration
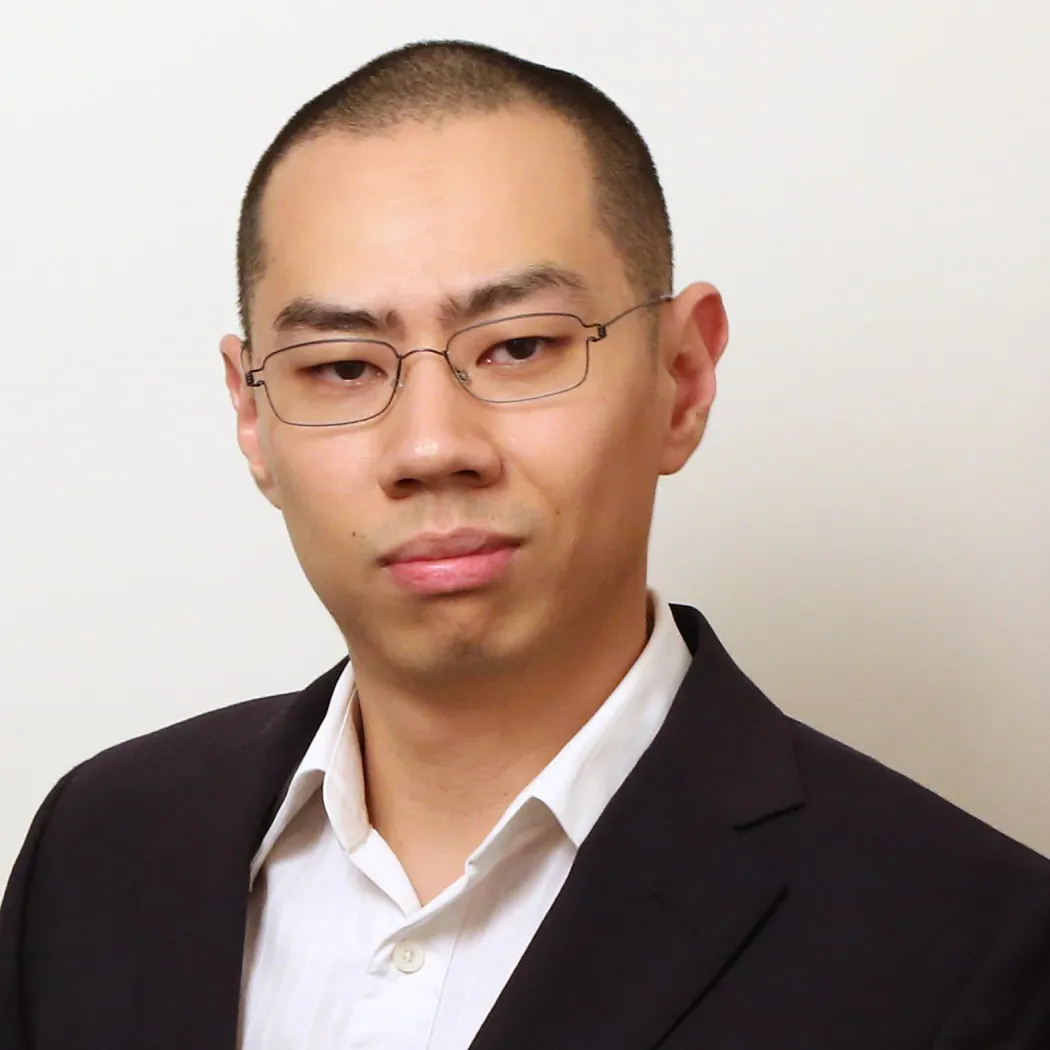
February/March 2025
by Frank Siu
The increasing adoption of artificial intelligence (AI) in investment management has sparked concerns over the potential for "crowding"—a scenario where many market participants rely on similar models, leading to correlated trades that erode performance benefits. Such fears evoke memories of the "Quant Meltdown" of August 2007, when widespread deleveraging resulted from the simultaneous unwinding of trades based on similar quantitative signals. The central question remains: does the widespread adoption of AI in stock selection and portfolio construction introduce costs that ultimately outweigh its advantages?
Arabesque's Portfolio Dragon platform seeks to integrate AI-driven forecasts into portfolio management. By processing tens of billions of data points through a sophisticated pipeline of supervised and unsupervised machine-learning model architectures, daily predictions of stock price movements are produced, enabling users to optimize their portfolios for improved performance.
To evaluate the feasibility of AI in portfolio management, we simulate its application across more than 10,000 globally listed equity funds, spanning a decade of holdings data. These funds include both actively managed mutual funds and exchange-traded funds (ETFs). Our findings indicate that AI improves the performance of 71% of these funds, with an average annualized return enhancement of 1.16%.
A key consideration, however, is whether the market impact and transaction costs associated with AI-driven trading erode these gains. Adopting a methodology similar to Ratcliffe, Miranda, and Ang (2017) and Siu (2020), we apply a nonlinear market impact model to assess the adverse effects of implementing the AI fund rebalancing trades. For simplicity, we assume portfolio rebalances are executed over a five-day period.
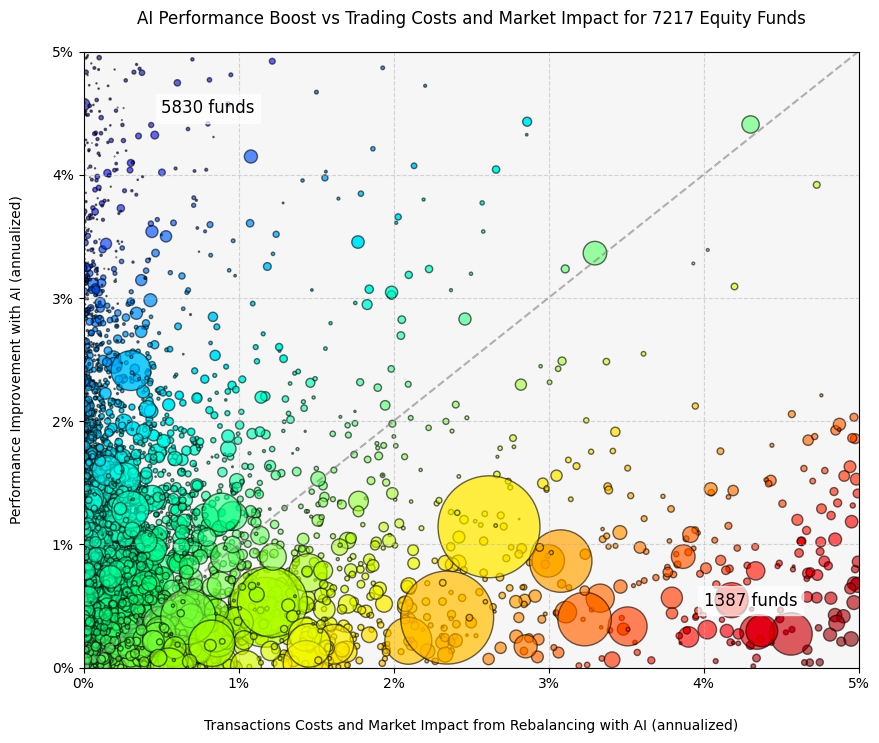
Focusing on the subset of funds where AI signals are able to enhance returns, we find that the vast majority (81%) remain profitable even after accounting for trading costs and market impact. Thousands of funds stand to benefit from an AI-driven return improvement of up to 3% per annum with minimal trading and impact costs.
A closer examination suggests that larger funds, as denoted by marker sizes proportional to assets under management (AUM), face greater challenges in capitalizing on AI forecasts. This result is unsurprising, given the greater market impact of their trades. However, these larger players may be well-positioned to leverage more sophisticated execution strategies—such as multi-period optimization trade scheduling models—to mitigate execution shortfall.
Aggregating the data by focus reveals further insights. While globally diversified and US-centric funds incur the lowest costs associated with AI integration, EMEA funds exhibit the narrowest gap between returns enhancements from AI and their market impact costs.
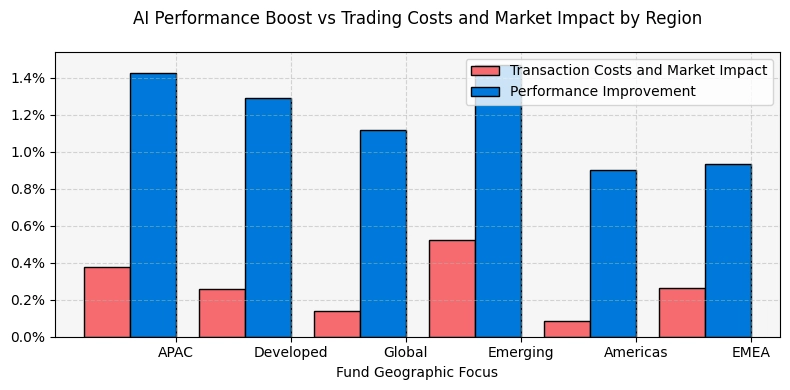
Finally, an assessment of scalability considers the extent to which funds can grow before their increased trading activity offsets the return advantages of AI integration. Our analysis suggests that, on average, funds could expand to three to six times their current AUM before "alpha" from our AI is fully absorbed by market impact.
For investors considering AI-driven portfolio enhancements, empirical results suggest substantial benefits, albeit with cost considerations that vary by portfolio characteristics. As such, we encourage users to experiment with the Portfolio Dragon platform, to more accurately assess the effect of AI integration into their portfolios, subject to highly customizable portfolio requirements.
References
Ratcliffe, Ronald, Paolo Miranda, and Andrew Ang. "Capacity of smart beta strategies from a transaction cost perspective." The Journal of Index Investing 8.3 (2017): 39.
Siu, Frank. "Where is There Room to Grow? Assessing the Capacity of Factor Investing Strategies." Qontigo/STOXX Research Paper. (2020)